We help you understand and utilise the power of your data and intelligently handle it
Robust analytics play a pivotal role in evaluating the efficiency and effectiveness of social welfare schemes. By providing relevant insights, analytics enables empirical policy decisions, eliminates ghost beneficiaries, detects identity fraud, correlates cause and effect, and refines policy interventions.
We offer opportunities to advance data analysis for public welfare. Our services streamline data collection, digitization, and curation, particularly focusing on urgent priorities. We integrate diverse data sources, even those not traditionally used together, creating models and predictions to support interventions. This facilitates a comprehensive evaluation of existing and historical policies, understanding their implications and enabling newer advances.
Functions under Data Analytics
Aggregation
We make technology assist you in your data collection
Utilizing technology for efficient data collection, we gather information from various sources based on your organization's needs. This includes collecting new data, utilizing previously stored data, or reusing data from other sources.
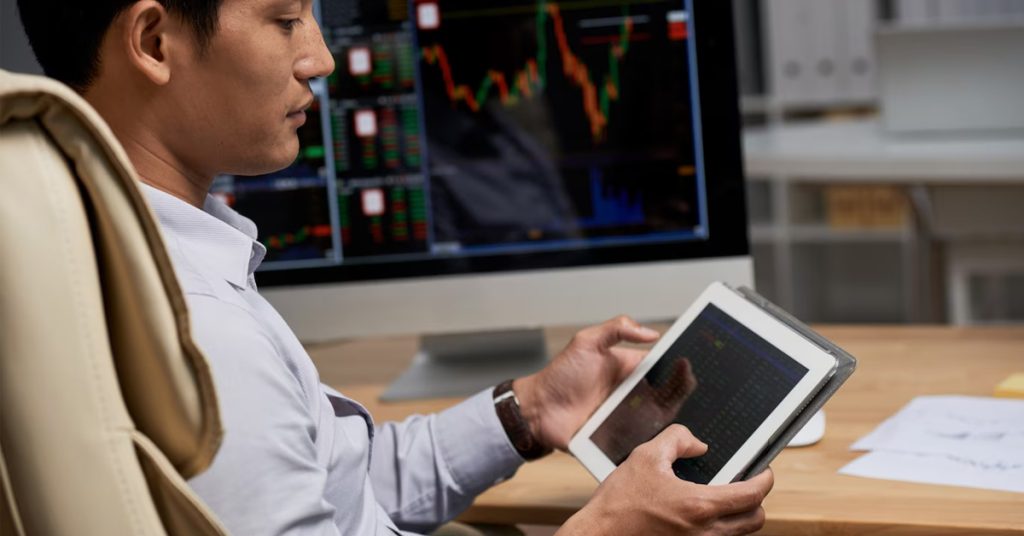
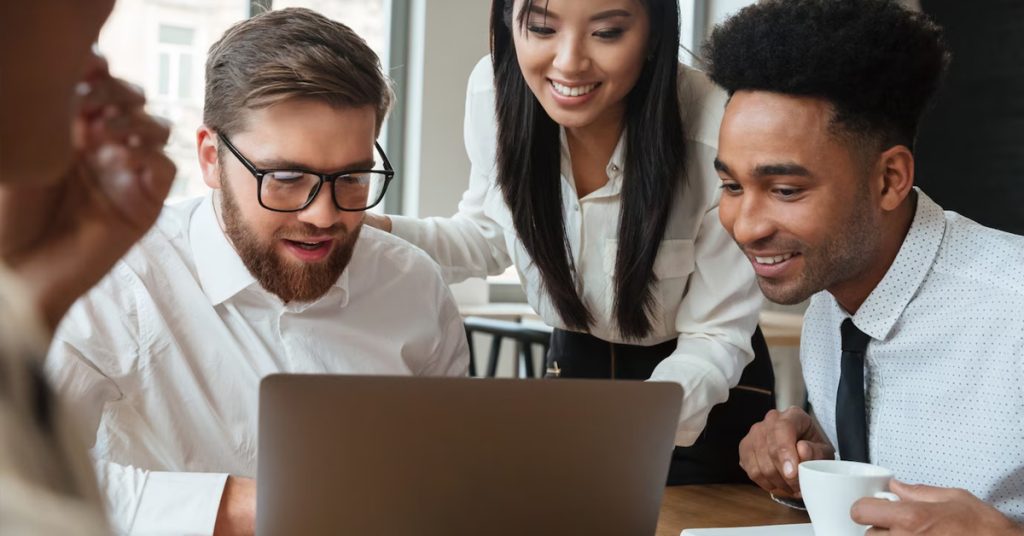
Processing
We help you see and analyse the data patterns
We assist in visualizing and analyzing data patterns. Often, collected data is voluminous and needs summarization before drawing conclusions. Whether numerical, interview transcripts, or descriptions, we organize the data for analysis. Our process includes error prevention and correction, ensuring the integrity of the data and avoiding potential issues.
Presentation
We sift through the vast data and find meanings
Navigating through extensive data, we extract meaningful insights. Employing exploratory data analysis techniques, we delve into the data to understand its underlying messages. This explorative process may lead to additional data cleaning or requests, forming an iterative approach. Descriptive statistics, such as averages or medians, are generated to enhance data understanding. Ultimately, we present the data graphically, providing additional insights into the information at hand.
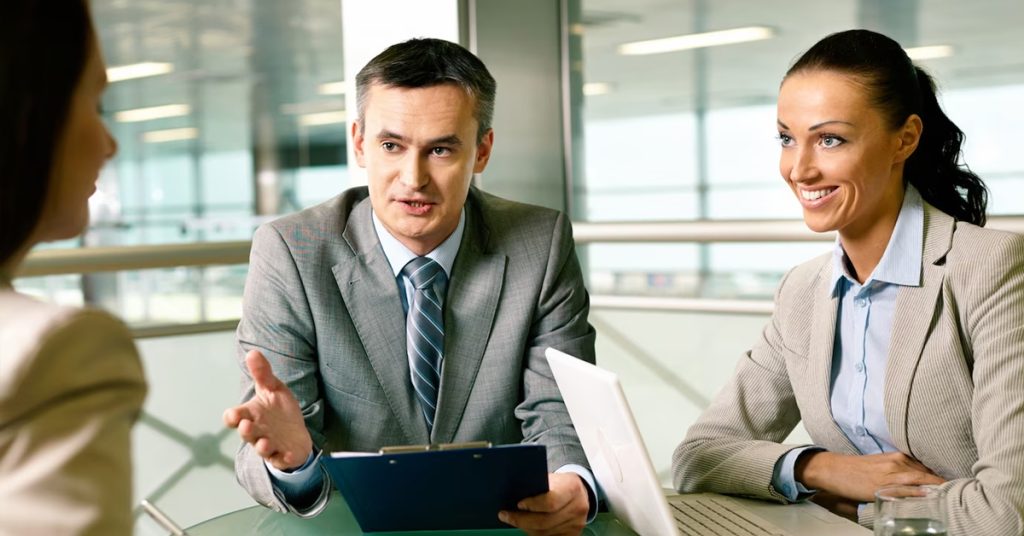